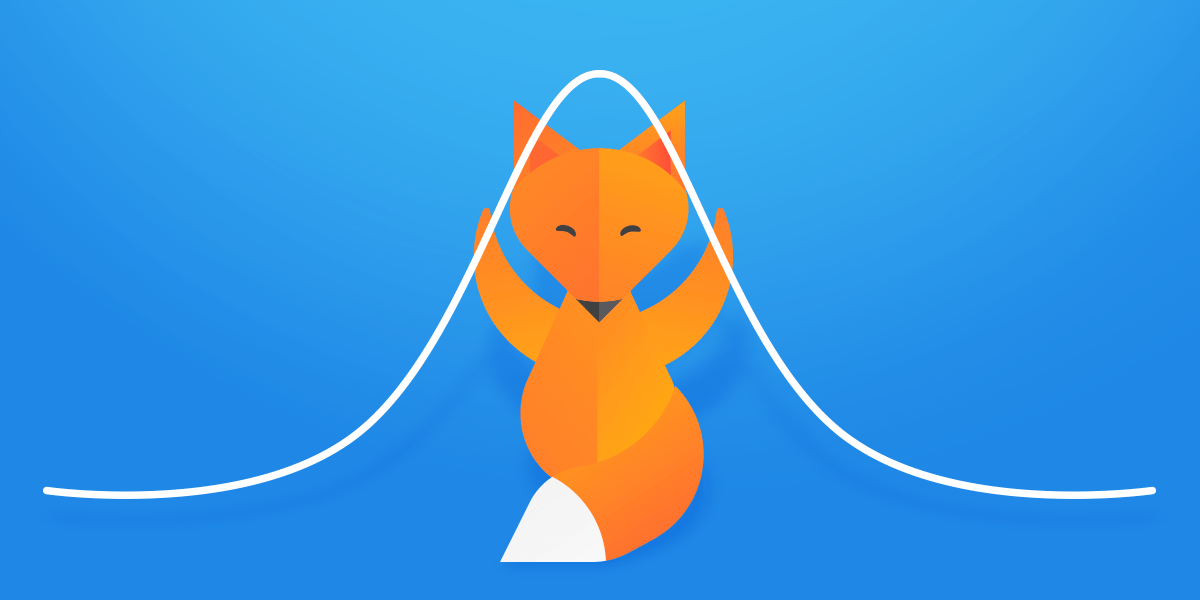
For many analytes, to be able to interpret a test result, we need to know what kind of results are typical in healthy persons. As these reference intervals depend on both the testing methods and the population, verifying reference intervals is an important task in laboratories. Some of our users have already used the quantitative comparison study for transferring reference intervals by setting range-specific goals for the existing reference interval and checking bias at both ends of the range. Yet, there hasn’t been a way to verify the transferred intervals.
The new Reference Interval study in Validation Manager addresses these needs. It enables you to establish reference intervals using nonparametric statistics as well as to verify existing or transferred reference intervals.
When establishing a reference interval, the statistical minimum for calculating the 95% reference interval is 39 samples, but at least 119 samples are needed to also calculate the related 90% confidence intervals. As you may remember, without confidence intervals, you cannot assess the reliability of your result, so 119 samples can be considered the minimum here. If you aim for some other coverage percentage than 95%, that will affect the number of samples needed. In this study, we are trying to collect a sample set that is representative of the entire population for which we are calculating the reference interval, so even larger sample sets are well justified — these are simply the statistical minimums.
Validation Manager uses the nonparametric method to calculate the reference interval from the result distribution. The results are ranked, and the limits of the reference interval are selected so that the same number of results at the lower and higher ends of the distribution will fall outside the reference interval, while a portion matching the coverage percentage will be within the reference interval. For example, for a 95% coverage percentage, the first and the last 2.5th percentiles are calculated as ranks corresponding with the lower and upper reference limits. If the rank is not an integer, the exact reference limit value is interpolated.
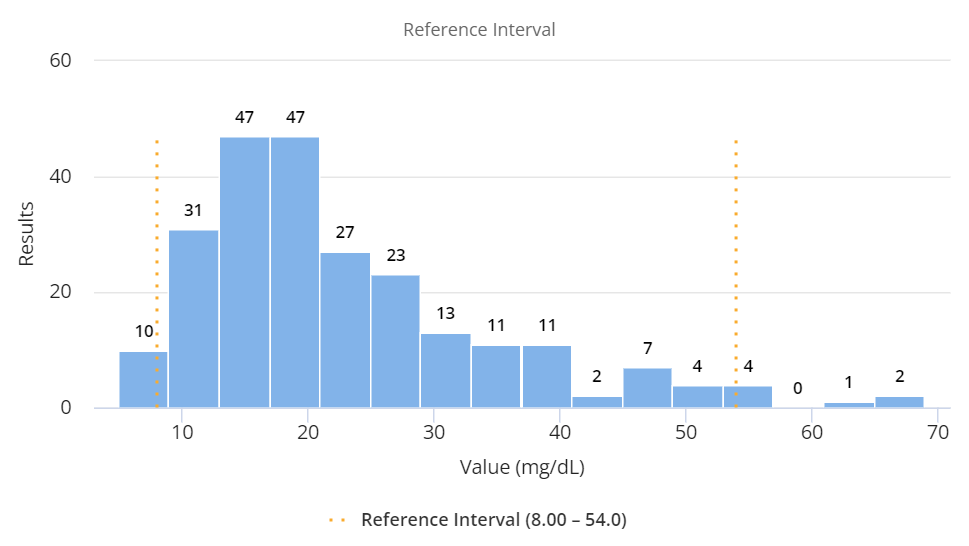
Histogram showing an example data set in a reference interval study
When verifying a reference interval, you don’t need quite as many samples. For example, you measure a set of 20 samples representing the population. If at most 10% of the results are outside of the reference interval, the reference interval is considered valid. If there are more results outside the reference interval, but less than 20%, then a second group of results can be tested to check whether the reference interval can be considered valid or not.
The workflow of the study is just as easy and nearly the same as it is in, for example, the Precision study. The biggest difference compared to other Validation Manager studies is that, in the verification protocol, the report evaluates the need for measuring the second group of results if the first group did not pass. In these cases, measure a second set of samples and import the results to the same study, and you’ll have information of both the results of the first and the second group on your report. This way, you don’t need to separately track how many groups you’ve analyzed for each analyte, as that information is automatically included in your report.
The new Reference Interval study can be found from the list of quantitative studies, and the same study works for both establishing and verifying reference intervals. At the moment, if you have multiple reference intervals for an analyte, for example in cases where the normal values in women differ from the normal values in men, you should create one study for women and another for men. We will improve this later to enable examining all population groups in one study, but for now you can only have one group for each analyte in a study.
If you have any feedback of the new study, please feel free to reach out to our user support team. We are always happy to hear of our users, and your input plays a key role in shaping our product development.
Accomplish more with less effort
See how Finbiosoft software services can transform the way your laboratory works.